the Creative Commons Attribution 4.0 License.
the Creative Commons Attribution 4.0 License.
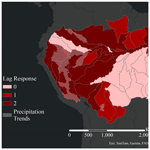
Riverine sediment response to deforestation in the Amazon basin
Anuska Narayanan
Sagy Cohen
John R. Gardner
The Amazon experiences thousands of square kilometers of deforestation annually with recent rates increasing to levels unseen since the late 2000s. These increased rates of deforestation within the basin have led to changes in sediment concentration within its river systems, with potential impacts on ecological functioning, freshwater availability, and fluvial and coastal geomorphic processes. The relationship between deforestation and fluvial sediment dynamics in the Amazon has not been extensively studied using a basin-wide, comparative approach primarily due to lack of data. In this study, we utilize a novel remote-sensing-derived sediment concentration dataset to analyze the impact of deforestation from 2001 to 2020 on suspended sediment in large rivers (>50 m wide) across the Amazon River basin. These impacts are studied using a lag-based approach to quantify the spatiotemporal relationships between observed suspended sediment and changes in land cover over time. The results show that large-scale deforestation of the Amazon during the 2001–2020 period are associated with significant changes in sediment concentration in the eastern portion of the basin. In the heavily deforested eastern regions, the hydrogeomorphic response to deforestation occurs relatively rapidly (within a year), whereas the less disturbed western areas exhibit delays of 1 to 2 years before responses are observable. Moreover, we observe that deforestation must be substantial enough to overcome the collective influences of human activities and natural sediment variations to result in a discernible impact on sediment concentration in large rivers. In 69 % of Amazonian major tributary basins with an immediate response, more than 5 % of the basin was deforested during the 2001–2020 period, while in 85 % of basins with lagged responses, less than 5 % of the land was cleared. These findings suggest severe implications for future sediment dynamics across the Amazon if deforestation is to further expand into the basin.
- Article
(8450 KB) - Full-text XML
-
Supplement
(1070 KB) - BibTeX
- EndNote
The Amazon River basin is the largest river system in the world, accounting for roughly one-fifth of global freshwater discharge (Callède et al., 2010) and supplying 40 % of the Atlantic Ocean's sediment flux (Milliman and Farnsworth, 2011). Though the Amazon is most often recognized for its rich biological diversity, the basin also performs a suite of ecosystem functions, such as local climate modulation and carbon sequestration (Foley et al., 2007). Despite its ecological importance, the Amazon experiences thousands of square kilometers of deforestation annually, with 2020 rates increasing to levels unseen since 2008 (Silva Junior et al., 2020). From 1975 to 2018, the Amazon experienced an accelerated rate of deforestation, with roughly 20 % (788 353 km2) of the Amazon deforested during this 43-year span (da Cruz et al., 2020). Deforestation alters the geomorphological, biochemical, and hydrological states of streams by decreasing land surface evapotranspiration and increasing surface runoff and river discharge, erosion rates (Horton et al., 2017), and sediment fluxes from land surfaces (Coe et al., 2011). For example, a 2003 study conducted within the Tocantins sub-basin (of the Amazon) noted a 24 % increase in mean annual water discharge and a 28 % increase in high-flow season discharge not attributed to changes in precipitation but rather by changes in land cover (Costa et al., 2003). A 2009 model simulation study using the same watershed determined that the increase in water discharge could not be solely attributed to climate variation (Coe et al., 2009), but two-thirds of the observed 25 % increase in discharge was instead attributed to deforestation that occurred during that period (Coe et al., 2011).
An intact forest cover is known to reduce runoff through various mechanisms such as canopy interception (Dykes, 1997), increased evapotranspiration (Ellison et al., 2011; Breil et al., 2021), and enhanced infiltration and soil moisture retention (Ellison et al., 2017; Ilstedt et al., 2007) and soil erosion control (Reubens et al., 2007; Flores et al., 2019; Veldkamp et al., 2020). Deforestation, however, reduces these capabilities by removing the protective canopy cover and vegetation roots that help to slow down surface water flow, increase infiltration, and stabilize the soil, leading to increased erosion rates (Veldkamp et al., 2020). Because of these impacts, it is suspected that the quantity of deforestation plays a significant role in the sediment response to land clearance. Likely, in areas with greater deforestation, the sediment response is generally more pronounced compared to areas with less deforestation. From a land–atmosphere approach, it is suggested that the impact of deforestation on the water cycle in the Amazon depends on various factors, such as the size and distribution of the deforested areas (D'Almeida et al., 2006). These factors can either increase or decrease the intensity of the water cycle in the region, depending on the specific deforestation scenarios. From a land surface hydrology perspective, this relationship may also apply; a larger cleared area exposes a greater amount of bare soil, which is more susceptible to erosion and sedimentation. In these cleared areas, rainfall, wind, and surface runoff can swiftly mobilize and transport the exposed soil into nearby waterbodies, leading to rapid sedimentation. Additionally, the increased fragmentation of forests into smaller patches in the Amazon (Broadbent et al., 2008) can further contribute to increased rates of soil erosion due to increased edge effects (Cardelús et al., 2020). For instance, the edges of forest patches are more exposed to environmental factors such as wind, rainfall, and sunlight. This exposure increases the vulnerability of the soil to erosion, as it is more susceptible to being dislodged and transported by wind and runoff. However, the presence of remaining vegetation and intact forests (in areas less deforested; Kroese et al., 2020; Wei et al., 2014), as well as reforestation, can mitigate erosion and sedimentation processes (Ouyang et al., 2013; Wei et al., 2009), thus slowing the sediment response in areas with less deforestation or smaller cleared patches.
Previous studies have observed significant increases in both sediment yield and concentration attributable to deforestation. While the majority of these studies have been limited in scale, focusing on smaller basins or study areas (Bringhurst and Jordan, 2015; Latrubesse et al., 2009; Ochiai et al., 2015; Maina et al., 2013; Maeda et al., 2008), recent advancements in satellite and remote sensing technologies have allowed for larger, global-scale analyses to take place (Dethier et al., 2022). Due to data constraints across the Amazon basin, studies previously conducted within the basin often adopt a “localized” examination on individual sub-basins. Little is known about how deforestation has impacted sediment concentration throughout the basin. While individual Amazonian sub-basins have been noted to exert significant increases in sediment due to deforestation, the methodologies employed in these studies may not be suitable for a basin-wide, annual examination of deforestation–sediment dynamics. Further, the diversity of methods used across these studies hinders direct comparisons of deforestation's impact between sub-basins, potentially overlooking broader patterns of deforestation-induced changes in sediment dynamics. For example, within the Suiá-Miçu River basin (located in the northeastern region of Mato Grosso) deforestation was observed to increase annual average sediment yields by 7 t ha−1 (Maeda et al., 2008). This was assessed by examining land cover changes during three periods in time (1973, 1984, and 2005) and using the Universal Soil Loss Equation (USLE) to identify changes in sediment yield. Although this study concluded that deforestation had resulted in significant increases in the sediment load, examining shifts between only three points in time introduces some uncertainty about these results. Further, the use of the USLE may not be the best choice in tropical climates as more than three quarters of all studies (conducted between 1977 and 2017) utilizing the USLE are focused on North America, Europe, or Asia; only 8 % of all studies during this period had been conducted in South America (Alewell et al., 2019). As the usability of the USLE is not well documented in the tropics, it may be inappropriate to apply these types of equations to complex tropical regions like the Amazon.
In the Magdalena River basin, located to the north of the Amazon River basin, deforestation in the Colombian Andes was observed to increase the basin's sediment load, with an estimated 9 % contribution from deforestation (Restrepo et al., 2015). In this study, the total area of deforestation was assessed for each of the Magdalena's sub-basins during the 1980–2010 period; these data were used to modify the anthropogenically induced erosion factor (Eh) of the BQART sediment modeling equation. By altering the Eh factor, Restrepo et al. (2015) observed an 11 % increase in model accuracy. Though this method allows for comparison of sediment load with and without anthropogenic input, it is based on a simple empirical model. Observations at the river basin scale are needed to quantify the sediment response to deforestation.
The number of readily available observational datasets within the Amazon has increased significantly in recent years (Crochemore et al., 2019); however, it is likely that basin-wide deforestation–hydrologic studies within the Amazon River basin remain limited due to a lack of high-quality water quality datasets. For example, Brazil's national hydrologic dataset, ANA Hidroweb (Water Resources National Agency, 2020), contains data on hundreds of river gauging stations. These stations collect water discharge and sediment concentration data throughout the country. However, sediment concentration measurements are spatially sparse and limited in long-term records for trend analysis. More than half of the stations lack data prior to 2007, and only a handful of stations contain observations for each year from 2001–2015. Other datasets, such as SO-HYBAM (Institut national des sciences de l'Univers, 2021), contain consistent, long-term observations and are updated frequently, but they only include 14 stations across the Amazon River Basin, limiting the scale to smaller catchments. Depending on their research goals, many studies in the Amazon use sediment modeling equations in place of in situ data (Maeda et al., 2008; Restrepo et al., 2015). As the Amazon River basin falls within the boundaries of eight different countries, it is difficult to compile the various national datasets available for a basin-wide analysis due to variations in data collection methods and the temporal availability of data.
Despite improvements to hydrologic models in recent years, using traditionally modeled data introduces some sources of error due to uncertainties in parameters, model structure, calibration, and input data (Moges et al., 2020). Recent advancements in remote sensing technologies have revolutionized our capacity to monitor and analyze environmental changes. The application of remote sensing in environmental sciences offers unparalleled advantages, including the ability to cover large spatial extents, provide long-term data coverage, and ensure data acquisition at lower costs compared to traditional methods. When coupled with machine learning algorithms, remote sensing data can be transformed into high-quality, comprehensive datasets (e.g., Global Forest Change Dataset; Hansen et al., 2013, World Settlement Footprint – WSF; Marconcini et al., 2020; and LandCoverNet; Alemohammad and Booth, 2020). This synergy is particularly effective in building high-quality river suspended-sediment concentration (SSC) datasets, enabling a more nuanced understanding of sediment dynamics over vast geographical areas and extended time frames. In this paper, we explore deforestation–sediment concentration dynamics across the 34 major tributary basins of the Amazon River basin using suspended-sediment data derived from new remote sensing observations. Applying a suite of statistical testing and comparative mapping, we explore the strength of the hydrogeomorphic response to deforestation, as well as response lags associated with deforestation magnitude. To overcome uncertainties associated with modeled data, sediment data in this study are derived from new remote sensing observations.
2.1 Study area
Despite its size (6 300 000 km2), the Amazon River Basin has a relatively homogenous climate due to its large tropical rainforest and its location situated along the Equator between 10° N and 20° S (Fig. 1). The basin is characterized as a tropical rainforest (Af by the Köppen–Geiger system) with average temperatures ranging between 24–26 °C throughout the year (Barthem et al., 2005). Typical of the Af climate type, the Amazon experiences large amounts precipitation annually. However, the spatial distribution of its receiving precipitation varies largely (1000–3600 mm) with annual rainfall exceeding 7000 mm along the southern Amazon–Andean transition line (Espinoza et al., 2015) and ranging from 1500 to 1700 mm in the drier regions of Roraima, Brazil, through the Middle Amazon to the state of Goiás (Barthem et al., 2005).
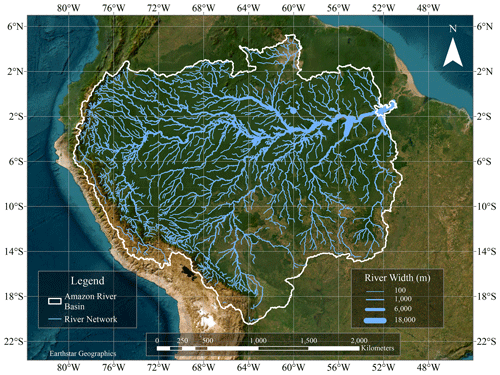
Figure 1The Amazon River basin with major streams and rivers. Line width symbolizes river width. Data used to produce river reach delineations are from the Surface Water Ocean Topography (SWOT) River Database (SWORD) centerlines (Altenau et al., 2021).
Topographic characteristics, such as hillslope steepness, can significantly influence soil erosion rates (Zhang et al., 2015); however, the majority of Amazon River basin is characterized by vast lowland areas. These lowland areas are relatively flat or gently sloping, with gradients that are generally not considered steep. Excluding the Andean region, the basin-wide median slope is 2.78° and carries an average slope of 5.32°. As a result, steep slopes and their associated effects, such as increased erosion and sedimentation, are less prevalent in much of the Amazon. Though hillslope steepness is recognized as a significant factor influencing sediment dynamics and is commonly used in modeling sediment transport, this study focuses on trends and relationships at the major tributary scale in the Amazon basin, and therefore we do not consider hillslope steepness in our analysis. The coarse analysis resolution used in this study, along with the predominance of lowland areas in the basin, limits the ability to capture fine-scale variations in hillslope steepness that may be present on a river reach-by-reach scale analysis.
2.2 Deforestation dynamics
The Global Forest Change (GFC) dataset is a remotely sensed, forest loss detection dataset developed by Hansen et al. (2013) in GEE. Using growing season imagery collected from the Landsat satellite series, the GFC dataset identifies changes in forest cover from the year 2000 to 2020 (v1.8) at a 30 m resolution. Forest loss is defined as a stand replacement disturbance, or a change from forest to non-forest (Hansen et al., 2013). In this context, the term “forest loss” does not equate loss caused exclusively by deforestation, as forest loss induced by natural disasters such as tornadoes, wildfires, and hurricanes is also included. Though the purpose of this study is to investigate the effects of deforestation on suspended-sediment load, the GFC dataset is used to identify areas affected non-natural forest loss (deforestation). In the Brazilian Amazon, 85 % of forest loss during the 2001–2013 period occurred as a direct result of deforestation (Deforestation in the Amazon, 2021). Other datasets, such as the Program to Calculate Deforestation in the Amazon (PRODES; Instituto Nacional de Pesquisas Espaciais, 2020) and the Palsar Global Forest/Non-Forest Maps from ALOS PALSAR (Shimada et al., 2014), were considered; however, these datasets did not have the spatial coverage or the temporal range desired for this analysis. The large spatial scale, temporal continuity, and high resolution of the GFC dataset remains unmatched to other forest-clearing datasets available, making it the most suitable choice for this study. Forest loss across the 2001–2020 study period, identified by the GFCC dataset, is shown in Fig. 2.
2.3 Sediment remote sensing dataset
Suspended-sediment concentration (SSC; mg L−1) concentration data were acquired using Landsat Collection 1 and machine learning using the methods described in Gardner et al. (2021, 2023) as Collection 1 was the best available product at the time. Landsat surface reflectance values were extracted over 18 401 river reaches (median length = 10 km) from the SWOT River Database or SWORD (Altenau et al., 2021) (ETM+) and Landsat 8 Operational Land Imager (OLI) using Google Earth Engine (GEE). Satellite imagery was captured mostly during the dry season, coinciding with the same period deforestation data are collected. While SSC data collected during the wet season may be preferable for studying deforestation-driven changes in sediment dynamics (most sediment production and mobilization typically occur during the wet season), using wet-season imagery was not possible due to high levels of cloud coverage. The SSC model was applied to this river surface reflectance database, which was processed and cross-calibrated across Landsat sensors to enable time series analysis (Gardner et al., 2021).
An Extreme Gradient Boosting (XGBoost) model was trained using 1112 matchups or satellite and SSC field observations that occurred within the same day or ±1 d following Ross et al. (2019). Field observation data used in the match ups were obtained from both gauging stations and grab samples spread throughout the basin (Fig. 3). Data from 121 gauging stations were sourced from ANA Hidroweb (Water Resources National Agency, 2020). ANA operates a network of automatic monitoring stations equipped with sensors to continuously measure various hydrological parameters, including sediment concentration, water level, flow velocity, and other water quality parameters. Additionally, grab sample data were collected from 14 SO-HYBAM gauging stations (Institut national des sciences de l'Univers, 2021). While the number of sampling locations is limited, these stations are strategically positioned throughout the Amazon Basin to represent diverse hydrological features such as main rivers, tributaries, lakes, and other waterbodies. These grab samples are collected manually by field personnel at regular intervals from the water surface (Institut national des sciences de l'Univers, 2021).
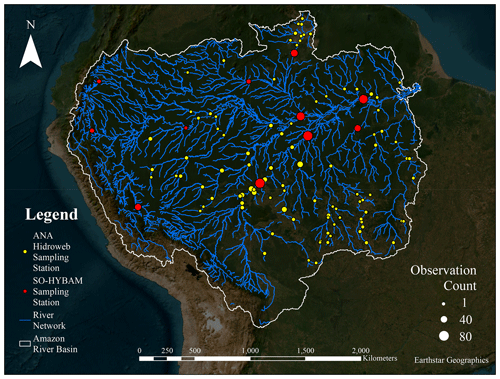
Figure 3Location of match up sampling points for training the XGBoost Model. The number of match up observations is indicated by the point size.
The model was built using methods described in Gardner et al. (2023) and includes forward feature selection, leave-time-out–leave-space-out spatial–temporal cross validation, and hyperparameter tuning (Meyer et al., 2018) to reduce overfitting and spatial–temporal bias and is validated on hold-out test data (Fig. 4a). XGBoost has four hyperparameters that were tuned using a grid search across all possible hyperparameter combinations with each parameter range centered around the default parameter values. The model performs well and can predict over an SSC range from 0.01 to 2500 mg L−1. This model has comparable error metrics to published models over large areas (Gardner et al., 2023; Dethier et al., 2020) and previous work in the Amazon (Yepez et al., 2018; Montanher et al., 2014). Specifically, the model exhibits a mean absolute error (MAE) of 32 mg L−1, a symmetric mean absolute percentage error (SMAPE) of 30 %, a percent bias (Pbias) of 11 %, RMSE of 58.7 mg L−1, and a very low relative error of 0.21 (Fig. 4a). In comparison, Gardner et al. (2023) reported a relative error of 0.59 for rivers in the USA, while Dethier et al. (2020) reported a relative error of 0.73 for rivers on a global scale. However, we focus on MAE and relative error as suggested by Seegers et al. (2018). While these studies are based on different regions and training datasets, they provide valuable benchmarks for evaluating the performance of the model in predicting SSC across diverse geographic and environmental settings. It should be noted that the SSC database generated focuses on surface concentration and may not accurately capture high SSC values due to factors such as cloud cover, sensor band saturation at high SSC, and a lack of high SSC field measurements for model training. However, it is important to emphasize that our primary goal is to assess relative changes in SSC over time and space. As such, the limitations inherent in remote sensing do not impact the validity of our results. Remote sensing remains the sole approach capable of generating consistent, spatially explicit, long-term (1984–2020) SSC observations across the Amazon Basin (see the Supplement for details of this method). Of the 17 182 river reaches in the Amazon, 10 932 reaches had at least 1 year of SSC data during the 20-year period (2001–2020). “Annual” values were computed by taking average SSC values of reaches with at least 6 samples during the dry-season period. Roughly 60 % of reaches with SSC data had at least 80 % of complete data (at least 18 years). Reaches ranged in length from 115 m to 20 km, with 58 % of reaches falling between 10 and 15 km in length (Fig. 4b).
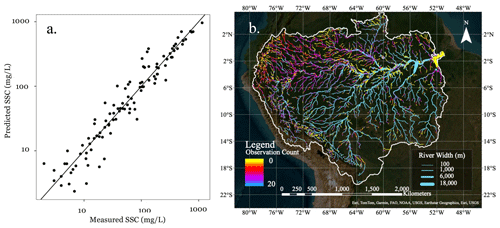
Figure 4(a) Validation plot of remote-sensing-derived (predicted) suspended-sediment concentration (SSC; mg L−1) vs. in situ SSC measurements (MAE = 32 mg L−1; relative error = 0.21; percent bias = 11 %; RMSE = 58.7 mg L−1) and (b) number of annually averaged SSC observations during the 2001–2020 period.
To prepare representative SSC data for trend and statistical analyses, SSC data were filtered based on data availability and reaches are aggregated by their major tributary basins. First, reaches with less than 10 years of SSC data are removed from the dataset. The remaining reaches are then grouped by their respective major and minor tributary basins defined by the Amazon Geographic Information System (GIS)-based river basin framework (Venticinque et al., 2016a). These basin delineations were chosen over other commonly used datasets, such as HydroBASINS (Lehner and Grill, 2013), due to its spatially uniform, multi-scale framework necessary for comparative statistical analyses.
While finer-scale analyses often offer detailed insights into small-scale variations and are useful in supporting local management goals, there are significant advantages to using large spatial analysis like major tributary basins in hydrogeomorphic analyses. First, major tributary basins provide a larger spatial analysis scale, allowing for a more comprehensive assessment of sediment dynamics across a wider area of the Amazon region. This broader perspective enables the identification of general trends and patterns in sediment concentration associated with deforestation. Second, major tributary basins tend to exhibit more consistent characteristics in terms of hydrological processes, land use patterns, and sediment transport. This consistency simplifies the analysis by reducing the variability introduced by smaller tributaries with unique geomorphological and hydrological characteristics.
Though most water quality studies tend to sample the basin outlet (e.g., Restrepo et al., 2015; Wasson et al., 2008; Diringer et al., 2019; Sweeney et al., 2004), there are several merits to using the basin average, rather than the basin outlet sediment concentration measurement in a deforestation–sediment study. For instance, the median basin value provides a more comprehensive and spatially representative measure of sediment concentration compared to measurements at the basin outlet. Sediment concentration can vary significantly within a river system, with different tributaries and sub-basins contributing varying amounts of sediment. Relying solely on the most downstream value could introduce bias and may not reflect the sediment conditions throughout the entire basin. Confluences with other rivers, changes in channel morphology, or the presence of reservoirs or dams can alter sediment transport patterns and influence sediment concentrations at specific locations. Further, the use of the median SSC and not the mean or maximum values provides resilience to extreme values. Extreme events such as floods or exceptionally dry periods can lead to transient spikes or depressions in sediment concentrations at specific points; however, the use of the median value reduces the influence of these extremes.
2.4 Precipitation and SSC trend analysis
To identify trends in SSC and precipitation over the 2001–2020 period, Mann–Kendall trend tests are performed over the Amazon's 172 minor tributary basins. Precipitation plays a significant role in shaping sediment trends by influencing sediment mobilization and transport (Renard, 1997; Wei et al., 2014). On the one hand, increasing trends in precipitation can result in more surface runoff, leading to increases in erosion and sediment mobilization (Armijos et al., 2020). Decreasing precipitation trends, however, can lead to reduced sediment transport due to limited surface runoff and decreased erosion rates (Ayes Rivera et al., 2021). Therefore, to limit the influence of precipitation, reaches within minor tributary basins bearing significant precipitation trends are excluded from the deforestation–sediment analysis.
To perform this analysis, daily rainfall data from the Climate Hazards Center at the University of California Santa Barbara is used (CHIRPS Daily: Climate Hazards Group InfraRed Precipitation; Funk et al., 2015). Daily precipitation values are summed for each month for each 0.05°×0.05° pixel, and the average summed value is calculated for each minor tributary basin. Precipitation trends are then calculated using these basin-averaged monthly precipitation totals. Reaches within minor tributary basins with significant trends are then removed from deforestation–sediment analysis (performed at the major tributary basin scale). Trends in SSC are then assessed at the minor tributary basin scale using a Mann–Kendall test on the median annual SSC measurement for each basin. By removing reaches with significant precipitation trends, the focus is narrowed to basins where the sediment response is primarily driven by deforestation, enabling a more focused assessment of the deforestation–sediment relationship.
2.5 Sediment response analysis
To assess the impact of deforestation on sediment concentration in the Amazon's 34 major tributary basins, we used a lag-based approach. It is suspected that the timing of sediment responses is closely linked to the intensity of deforestation. Specifically, in basins with higher levels of deforestation, a relatively rapid hydrogeomorphic response is expected. Conversely, in less disturbed (more pristine) basins, a delayed response is anticipated. Therefore, a time-lagged cross-correlation (TLCC) analysis is used to identify lags in sediment response to deforestation. TLCC analyses are frequently used to identify lag responses in discharge, deposition, and water quality within watersheds (Yang et al., 2023; Kovacic and Nataša Ravbar, 2010; Durin et al., 2023; Chen et al., 2014). These types of correlation analyses are useful for determining the amount of time required to pass for a response to occur. For example, given two phenomena differing by an unknown amount of time, one can use a cross-correlation to determine how much one variable must be shifted along the x axis to align with the other. Essentially, the shift is identified using the peak Pearson correlation (r). For each major tributary basin, the median sediment concentration is calculated for each year in the 2001–2020 period (n=20). This median value is then correlated with the percentage of deforestation that occurred in these corresponding years to quantify the co-variation in sediment and deforestation temporal trends. We use the annual deforestation percentages as a “stationary” predictor variable and test lag responses in the median annual SSC concentration. We confine the results presented here to a maximum of a 2-year lag based on our preliminary analysis, which found no significant co-variation when using three or more year shifts.
After identifying response lags within the Amazon's major tributary basins, two statistical tests are used to explore the relationship between deforestation and identified lags. A Kruskal–Wallis (K–W) test is used to identify significant variations in deforestation intensity between all three lag groups, while a Fisher's exact test is used to test for significant association between the deforestation intensity (categorized as high or low) and the presence of response lags. These tests serve distinct but complementary purposes in understanding the relationship between deforestation and response lags. While the K–W test provides a broad view of deforestation intensity patterns across various lag groups, the Fisher's exact test focuses on the specific linkages between deforestation intensity and response lags. To conduct the K–W test, basins are first separated by their respective lag groups (based on the TLCC analysis). Subsequently, the total percentage of deforestation within each basin during the 2001–2020 period is calculated, forming the basis for the K–W test. For the Fisher's exact test, basins are categorized into two primary groups based on deforestation intensity: high deforestation, consisting of basins with over 5 % of their area deforested over the 2001–2020 period, and low deforestation, consisting of basins with less than 5 % deforested. Likewise, basins are grouped into two categories based on their lag response: those with a lagged response and those with an immediate response. The Fisher's exact test is then applied to these groupings to examine potential associations.
To quantify the influence of deforestation on sediment concentration annually, we compare annual SSC and deforestation using a correlation analysis. Because sediment concentration can vary significantly across different basins, concentration values are normalized by measuring their deviation from the mean (i.e., standard anomaly) using Eq. (1) below. While normalized values are commonly employed in climate studies to compare diverse phenomena like temperature and precipitation (American Academy of Actuaries, 2016), in this context, we use normalization to investigate how deforestation affects concentration changes on an annual basis between different lag groups. For the analysis, annual normalized SSC values (SSCn) are computed for each basin. The basins are then categorized into their respective lag groups, and SSCn values are synchronized with their expected deforestation year before conducting a correlation analysis. This approach, based on lag groups, acknowledges the potential variation in sediment response dynamics between basins exhibiting rapid responses (within a year) and those showing lagged responses (with 1 to 2 years). Further, it allows us to determine if the impact of deforestation on SSC remains consistent between different lag groups.
where SSCn,μ is the normalized SSC for year μ, is the 2001–2020 average SSC sediment concentration, SSCμ is the concentration value for year μ, and SSCσ is the 2001–2020 standard deviation. Positive and negative SSCn indicate above and below 2001–2020 average SSC, respectively.
2.6 Data scaling
Within this study, deforestation and sediment concentration patterns are examined at the major tributary basin analysis scale, while precipitation and SSC trends are isolated at the minor tributary basin scale. Initial trend and correlation assessments were conducted at the river reach level for precipitation, SSC, and deforestation. However, these analyses often resulted in inconsistent findings that lacked spatial uniformity. For example, high levels of variability in SSC trends were often noted between river reaches of the same river (Fig. S3). Similarly, an assessment on lags performed at the minor tributary basin aggregation scale yielded similar, non-uniform results (Fig. S4). Though precipitation and SSC trends were observable at this scale and demonstrated significant spatial consistency (meaning basins with significant trends tended to be close to each other), relationships between SSC and deforestation remained inconsistent. This contrasts examinations at the larger major tributary basin scale, which revealed clearer spatial patterns of SSC–deforestation relationships. These outcomes suggest that at finer scales, local variations and fluctuations likely carry a significant influence on sediment concentration leading to a high sensitivity to small-scale factors.
3.1 Temporal trends in precipitation and sediment
Significant trends in precipitation between 2001 and 2020 (p<0.05) were observed in the western portion of the Amazon near the Andes (Fig. 5a). A total of 12 minor tributary basins were noted as having increasing precipitation trends, while one basin had a decreasing trend. Notably, significant SSC trends and patterns were identified across the Amazon basin. In the eastern portion of the Amazon, several sub-basins showed significant increases in SSC trends (Fig. 5b), coinciding with relatively high deforestation rates (Fig. 2). In the north, a separate cluster of basins with increasing SSC trends is also observed. While deforestation does occur in this region, its rate is not nearly as high as in the east of the Amazon basin (Fig. 2).
3.2 Time-lagged cross correlation
The time-lagged cross-correlation analyses revealed significant patterns in lags throughout the Amazon. Prior to applying the lags, the Pearson's correlation (between median annual SSC and annual percentage of the basin deforested) appeared somewhat weak in many of the Amazon's sub-basins, particularly in the west where deforestation is limited (Fig. 6a). In fact, negative correlations were observed in many of these basins, meaning that increases in deforestation were associated with decreases in sediment concentration. However, after adjusting for lags, the correlation between deforestation and SSC increased throughout much of the basin (Fig. 6b). This indicates that the observed negative correlations were likely a result of a misalignment in the hydrogeomorphic response to deforestation. Although this adjustment improved the temporal alignment for many of these basins, five basins continued to have a negative correlation following the lag adjustment.
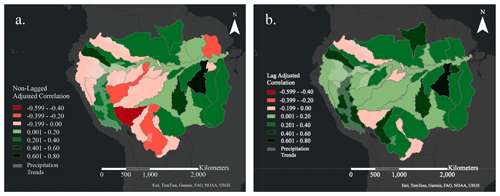
Figure 6Correlation coefficients prior to applying lags (a) and correlation coefficients after applying lags (b).
Mapping the optimal lag for each sub-basin elucidates a distinct spatial pattern within the Amazon (Fig. 7). There is a notable concentration of basins with zero lag in the eastern regions, aligning with the historical trend of intensive deforestation and human settlement primarily occurring in these areas. In contrast, basins that exhibit a lag of 1 or 2 years are characterized by lower levels of deforestation. The contingency table (Table 1) provides a clear breakdown of these observations, quantifying the frequency of high vs. low deforestation rates in relation to the presence or absence of a lag response. Significantly, about 61 % of the basins analyzed have a lagged response, and within this group approximately 85 % have undergone minimal deforestation, defined as less than 5 % of the basin area. On the other hand, 69 % of the basins with no lagged response are subject to higher deforestation rates, exceeding 5 % of their area. These patterns indicate that heavily deforested basins are more likely to exhibit direct changes in sediment concentration vs. the more “pristine” basins.
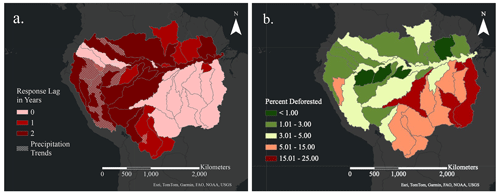
Figure 7Identified lag response (in years) present in each major tributary basin (a) and total percentage of each major tributary basin deforested in the Amazon from 2001–2020 (b). River reaches falling within the precipitation zone (sig. precipitation trends) were excluded from the basin lag analysis.
Table 1A 2×2 contingency table showing response lag presence vs. deforestation. Data are reported in terms of percent. Sub-basins with “low” levels of deforestation (<5 % of basin deforested) carry the majority of lags within the Amazon, while basins with “high” levels of deforestation (>5 % of basin deforested) tend towards an immediate hydrogeomorphic response.
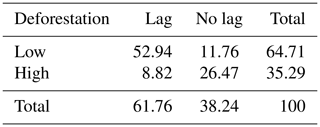
The Fisher's exact test revealed significant associations between deforestation (high vs. low) and lag presence (Table 2). Basins with large amounts of deforestation (>5 %) were less likely to exhibit lags in SSC response. Similarly, basins with less deforestation (<5 %) were more likely to exhibit lags. Further, significant results were observed when performing a one-tailed (right) Fisher's exact test, indicating a strong association between the absence of lags and high deforestation rates. These results indicate that deforestation has a strong, direct impact on sediment dynamics, with more intensive deforestation activities leading to quicker hydrogeomorphic responses in the affected basins.
Table 2Results of the association tests between high and low deforestation and lagged and non-lagged basins.
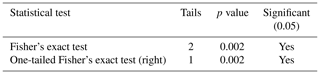
Prior to performing the Kruskal–Wallis Test, a modified box plot and Grubb's test were used to identify any basins with unusual levels of deforestation (outliers). The Curuá Una sub-basin stood out as having an unusually high amount of deforestation despite its small size. Curuá Una, approximately 83 % smaller than the average basin, had 23 % of its area deforested from 2001–2020. Due to its small size and the significant impact of even a small amount of deforestation, Curuá Una was excluded from the analysis. Upon categorizing the data into different lag groups, another outlier was identified: the Huallaga River basin. While the Huallaga, like many other Amazon basins, has experienced substantial deforestation over the past 2 decades, the nature of land use following clearance sets it apart from other basins. Unlike the predominant cattle ranching and soy cultivation that drives deforestation in most Amazon basins, deforestation in the Huallaga is primarily driven by coca cultivation for cocaine production (Van Dun, 2009; Pruett, 2014). The land in the region is promptly replanted after deforestation instead of being converted to pasture. This distinction suggests that the hydrologic response to deforestation in the Huallaga differs from that of other basins. As a result, the Huallaga basin was also excluded from the sample to account for these dissimilarities.
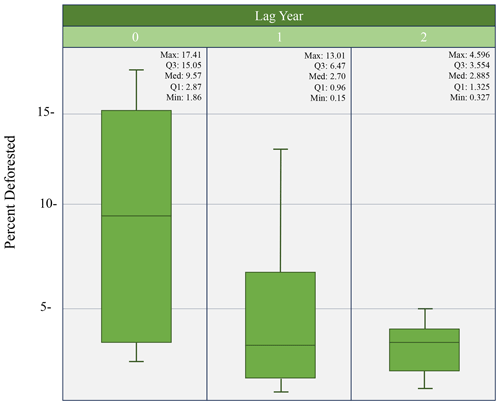
Figure 8Percent of each basin deforested by lag year. Two outlier basins, the Curuá Una and the Huallaga basins, were removed from L1 (Curuá Una, 23 %) and L2 (Huallaga, 10 %).
The Kruskal–Wallis Test demonstrated significant variations in the total deforestation percentages (2001–2020) among the different lag groups (p value of 0.0209, H statistic of 7.734). Sub-basins with 0 years of lag (L0), indicating a more immediate response, exhibited a higher average percentage of deforestation compared to sub-basins with lags of 1 (L1) or 2 (L2) years (Fig. 8). Similarly, sub-basins with a lagged response of 1 year displayed a greater average percentage of deforestation than sub-basins with 2 lag years. Table 3 provides a summary of these observations. To ensure that these differences are attributed to deforestation rather than inherent basin characteristics, a similar analysis was conducted considering basin size and the number of river reaches falling within the basin. However, no significant differences in deforestation were found through these analyses, suggesting that the temporal response to deforestation is strongly contingent on the extent of deforestation taking place. This further implies that deforestation intensity directly influences the timing of sediment response, with more immediate hydrological alterations occurring in basins experiencing higher deforestation rates.
3.3 Correlation analysis
The correlation analysis between the normalized SSC for each year (SSCn,μ; Eq. 1) and lag-adjusted annual deforestation percentages provided interesting insights into deforestation–SSC relationships across different lag groups (Table 4). As expected, the L0 group showed the highest overall correlation (r=0.184; Fig. S5) compared to the L1 (r=0.051; Fig. S6) and L2 basins (r=0.116; Fig. S7). Similarly, the average correlation within each lag group varied strongly, with the L0 basin grouping carrying the highest average correlation (r=0.335), followed by L1 basins (r=0.260) and L2 basins (r=0.115). These findings suggest that the geomorphic response to deforestation is highly specific to each sub-basin (i.e., no strong association) except for in regions with a relatively high intensity of deforestation (L0).
To further explore these relationships, a Mann–Whitney U test is used to identify differences in deforestation rates between years characterized by positive and negative SSCn,μ values. This test was performed separately within each lag group to examine how response lags might influence the impact of deforestation on sediment dynamics. SSCn,μ were grouped into a positive and negative years (above and below mean, respectively). A Mann–Whitney U test was then performed within each lag group to assess the differences in deforestation between positive and negative SSCn,μ. Not surprisingly, significant differences in deforestation patterns were observed within the L0 group (p=0.002; Table 5). In these sub-basins, which exhibit an immediate response to deforestation, years with higher-than-normal sediment concentration (positive SSCn,μ) were strongly associated with elevated deforestation rates. Similarly, years with lower sediment concentration (negative SSCn,μ) were strongly associated with lower deforestation rates. In contrast, no significant differences in deforestation were observed in the L1 and L2 groups (p=0.344 and 0.155, respectively). These results suggest that the impacts of deforestation on SSC are most pronounced in basins without a lagged response, while the relationship becomes less significant or more complex in basins with lagged responses. Despite its unusual nature, this finding is not surprising. In basins with high deforestation rates, deforestation is expected to have a more significant impact on sediment concentration compared to basins with low deforestation rates. In the latter case, sediment dynamics are likely to be more influenced by other factors such as damming, mining, agricultural practices, and urbanization. Likewise, these factors may cause the discernable signal of deforestation induced sediment to be “washed out” over time. These results further suggest a nonlinear or perhaps threshold-dominated relationship.
In a basin as large as the Amazon, it is difficult to make definitive, basin-wide statements on deforestation–sediment relationships. In some Amazonian sub-basins, these relationships appear very clear, evident by the results of the time-lagged cross-correlation (TLCC) analysis (Fig. 6b). In other sub-basins, however, these relationships are unclear with weak or negative correlations present. From the correlation analysis, it is suggested that the strength of SSC–deforestation relationships is tied directly to deforestation intensity (Tables 4 and 5). The stronger correlation between SSC anomalies and deforestation found in basins with no lags (L0; r=0.184, p=0.004) can be attributed to the stronger presence of deforestation. In contrast, basins with lag responses (L1 and L2) display a diminished correlation (r=0.051, 0.116, p=0.508, 0.089, respectively). This decline in correlation strength likely arises from the lower occurrence of deforestation in lagged basins (Fig. 8), allowing external factors such as anthropogenic activities and natural variations to exert a more dominant influence on SSC dynamics. Moreover, as time elapses between deforestation and response, the signal tends to be “washed out”, diminishing its clarity and detectability.
Similarly, the results of the Fisher's exact test (p=0.0002) suggest that the presence of SSC response lags is strongly tied to deforestation intensity. In basins characterized by significant deforestation (>5 % deforested), there is a decreased tendency for lags to be present; conversely, in basins with relatively little deforestation (<5 % deforested), there is an increased tendency for a lag response to exist. These patterns align with the findings from the K–W test (p=0.0209), which suggest that deforestation intensity may influence the number of lag years. These findings suggest two important insights regarding the impact of deforestation on sediment concentration. First, on a broad scale, a significant level of deforestation is required to generate an immediate impact on sediment concentration. As forest and vegetation landscapes experience degradation and fragmentation, their ability to buffer and mitigate soil erosion weakens. Simultaneously, intense deforestation practices lead to increased soil erosion, resulting in a greater amount of sediment available to the river system. This creates a compounding “snowball” effect, where sediment delivery and deposition become amplified. Second, the impact of deforestation on sediment concentration is not solely determined by the extent of deforestation. Other factors, such as damming, mining activities, and basin characteristics, can attenuate the relationship between deforestation and SSC. These factors may make the relationship less apparent or even non-existent.
For example, mining activities generate large volumes of exposed soil and sediment, not only through land clearance but also through excavation, blasting, and ore processing. The loosened soil and tailings can then be easily transported by rainfall and runoff into nearby rivers and streams. Soil characteristics are another potential factor that can influence sediment dynamics. High cohesion is a basin's dominant soil groups, for example, may result in reduced transportability. Consequently, even with deforestation and the removal of vegetation cover, the cohesive nature of the soils can impede sediment erosion and transport, contributing to a negative response in sediment concentration. Alternatively, damming can have a significant impact on sediment dynamics by acting as a sediment trap, capturing and accumulating sediments upstream. This process effectively reduces the downstream transport of sediments, leading to a decrease in sediment concentration immediately downstream (Zhou et al., 2020; Moragoda et al., 2023). To observe a noticeable and immediate impact of deforestation on sediment concentration, the magnitude of deforestation must be substantial enough to surpass the influence of these other factors. In other words, deforestation must be significant to overcome the combined effects of human activities and natural sediment variations to produce a discernible influence on river sediment concentration.
Other factors, such as basin size, can also affect the discernable influence of deforestation on sediment. In previous studies, strong relationships between deforestation and sediment are observed within relatively small river basins. For example, in New Zealand's Waipoua River system, which encompasses an area of 1987 km2, Kettner et al. (2007) observed a 6-fold increase in suspended-sediment discharge at the river outlet due to deforestation. In Wisconsin's North Fish Creek (drainage area of 122 km2), deforestation and human settlement was observed to increase sediment to 4–6 times pre-settlement rates (Fitzpatrick and Knox, 2000). In larger catchments, however, the influence of deforestation on sediment appears to be much lower as more variability is introduced into the relationship. For example, within Spain's Ebro River basin (85 530 km2), long-term anthropogenic land use was revealed to increase sediment by 35 %, from 30.5 to 47.2 Mt yr−1 over a 4000-year period (Xing et al., 2014). In the Magdalena River basin (273 459 km2), Restrepo et al. (2015) observed a 9 % increase in sediment load attributable to deforestation. Our observations within Amazonian major tributary basins align with these overall trends. The majority of basins within our study were each greater than 100 000 km2; therefore, it is to be expected that the discernable influence of deforestation on sediment dynamics may exhibit greater variability or attenuation compared to smaller basins. Some studies have found that the influence of land use and land cover change on runoff (Blöschl et al., 2007) and discharge (Zeilhofer et al., 2018; Rodriguez et al., 2010) decreases with watershed size. Likely, in the case of sediment transport, transport processes, such as deposition and dilution of the deforestation-sourced sediment, are magnified at these larger basin scales. The increased occurrence of these processes allows for large basins to have a greater buffering capacity and therefore produce a small sediment delivery ratio (Walling, 1983, 1999). These relationships likely result in the observed variable relationship strengths (Fig. 6).
While the concept of lagged responses in sediment yield due to land disturbances is not a novel concept (Owens et al., 2010), examinations of the timing of these impacts are somewhat limited. For example, while there have been many examinations of the long-term and future impacts of land clearance on sediment quantity and quality (Xing et al., 2014; Talib and Randhir, 2023; Kreiling et al., 2020) and soils (Veldkamp et al., 2020), studies specifically investigating the immediate vs. delayed effects of deforestation on sediment transport are somewhat limited (Ochiai et al., 2015). Studies addressing lag effects within river basins often focus on lags in precipitation and runoff (Zeilhofer et al., 2018) or discharge (Costa et al., 2009; Coe et al., 2011), lags in deforestation and carbon emissions (Rosa et al., 2016), or lags in water quality responses to land treatments (Meals and Dressing, 2008). The lag relationships identified within this study offer a unique insight into the temporal dynamics of sediment transport and suggest that consideration of deforestation intensity is a key factor in understanding the true impact of land disturbances. However, additional research on these temporal dynamics is necessary to produce a comprehensive timeline of sediment dynamics following land disturbances.
Despite the valuable insights gained from this study, it is important to acknowledge the limitations associated with data aggregation and resolution, both spatially and temporally. This research primarily focuses on the impact of deforestation on sediment concentration within the Amazon's major tributary basins. While this approach offers a broad, comprehensive view of sediment dynamics, it overlooks finer-scale variability and localized effects within each basin. Consequently, the findings may not fully encapsulate the complex and variable nature of deforestation-induced sediment dynamics at finer scales. Attempts to explore relationships using finer aggregation scales, such as river reach or minor tributary basin levels, did not yield easily discernible trends and patterns across the basin. This challenge may be linked to the use of coarse temporal measurements (annual), which might have obscured finer-scale dynamics operating on shorter timescales. Previous works have observed that the most substantial impact of deforestation on the sediment delivery ratio usually occurs immediately after the disturbance event (Lal, 1997; Ochiai et al., 2015). Therefore, the use of annually aggregated data may obscure fine-scale temporal patterns, such as seasonal fluctuations and the influence of specific disturbance events. Consequently, this temporal aggregation may hinder our capacity to establish direct cause-and-effect relationships between deforestation and sediment concentration at specific locations within the Amazon basin. Future work could explore attributing changes in SSC to specific anthropogenic activities and regenerative processes in the Amazon. For example, attribution methods previously established to examine controls of sediment flux in other basins such as the Jialing River basin (Zhou et al., 2020) and the Yellow River basin in China (Wang et al., 2016) could be adapted to the Amazon. Similarly, the use of land cover datasets designed for Amazonian-type landscapes such as MapBiomas (Souza et al., 2020), which covers Brazil, may unveil more regionally specific relationships than when using global land classification algorithms. While this study underscores significant connections and relationships between deforestation rates and sediment concentration, attributing the observed changes solely to deforestation requires more detailed data and comprehensive analyses.
Another limitation of this study relates to the use of concentration values over sediment transport values (flux; kg s−1) to assess deforestation–sediment relationships. In regions characterized by extensive deforestation, it is possible that the presence of larger discharge values (which inversely decrease SSC) act as a masking factor, potentially obscuring a more substantial impact of deforestation on sediment dynamics than what is observed in this study. Deforestation has been observed to increase surface runoff in many parts of the world (Guzha et al., 2018; Potić et al., 2022; Zhao et al., 2022), leading to the dilution of SSC, and making it challenging to discern the true extent of the influence of deforestation on sediment concentration. While these observations are often more present in water-limited watershed (Zhang et al., 2017), and observations of this phenomenon have been somewhat limited in the Amazon (Lucas-Borja et al., 2020; Voldoire and Royer, 2004), there may be overlooked decreases in SSC associated with increasing discharge values. Utilizing sediment transport values may unveil an even more profound effect of deforestation on sediment dynamics in these heavily deforested areas not elucidated in this study. To better capture the true extent of deforestation's impacts, future examinations on deforestation–sediment dynamics in the Amazon should consider using both SSC and discharge data in their analyses.
Despite these limitations, this research provides valuable insights into the complex nature of deforestation–sediment relationships within the Amazon. Though associations between deforestation and suspended-sediment concentration are not uniform across the Amazon basin, this work suggests the impact of deforestation is likely influenced by three main factors: (1) the extent of deforestation itself, (2) the presence of external sediment-altering factors, and (3) the specific environmental context of each sub-basin. As deforestation intensifies, the impact on sediment concentrations is likely to become more pronounced. However, delays in response within less deforested basins may indicate the presence of natural buffers that mitigate sediment impacts and a stronger influence of factors not directly related to deforestation on sediment dynamics.
From this study, it is evident that deforestation plays a significant impact on sediment dynamics at the large-basin scale across the Amazon, particularly in basins with intense deforestation. The hydrogeomorphic response to deforestation was observed to be relatively rapid (within a year) in highly disturbed basins, while a 1- to 2-year lagged response was observed in less disturbed basins, potentially due to the influence of other factors such as natural sediment variations, human activities, and soil characteristics. We find that the impact of deforestation on sediment concentration is directly tied to the magnitude of deforestation. For deforestation to have a detectable influence on sediment concentration in large rivers, it needs to be substantial enough to surpass the combined effects of human activities and natural sediment variations. Further, increases in sediment concentration were found to be positively correlated with the magnitude of deforestation rates, emphasizing the importance of considering the extent of deforestation when assessing its impact on sediment concentration.
These findings have potential implications for environmental management and policy development in the Amazon region. While this study does not directly attribute the observed increases in sediment concentration in eastern Amazonia to deforestation, based on our results it is likely that if deforestation expands deeper into the Amazon, the fluvial response can rapidly intensify. This underscores the importance of implementing sustainable land use practices to mitigate soil erosion and maintain Amazonian River systems. Incorporating finer-scale spatial and temporal data to capture the localized variations and transient dynamics of sediment concentrations following deforestation events will potentially allow for better understanding of the specific drivers and processes involved. This expanded knowledge can help identify critical areas where interventions are needed to mitigate the negative impacts of deforestation on riverine sediment dynamics and associated ecological consequences. As anthropogenic activities continue to alter the Earth system, understanding both the intended effects and unintentional consequences of these activities are vital to sustaining a future on Earth.
The code used to generate SSC data is available at https://doi.org/10.5281/ZENODO.8377852 (Gardner, 2024).
This study utilizes several publicly available precipitation and forest loss data and river reach and basin shapefiles. Precipitation data are from the Climate Hazard Center UC Santa Barbara CHIRPS dataset and can be accessed at: https://catalogue.ceda.ac.uk/uuid/4e53c2aee3fe44e7aa107c163696d2e7 (Climate Hazard Group, 2018). The forest loss data used in this research are obtained from the Hansen Global Forest Change dataset (Hansen et al., 2013). The SWOT River reach data utilized in this study can be obtained from the following source: https://doi.org/10.5281/ZENODO.4917236 (Altenau et al., 2021). Additionally, shapefiles for the Amazon Aquatic Ecosystem Spatial Framework are available for download through the SNAPP Western Amazon Group and can be accessed via the following link: https://doi.org/10.5063/F1BG2KX8 (Venticinque et al., 2016b).
The suspended-sediment concentration (SSC) data, RivSed-Amazon, is available at https://doi.org/10.5281/ZENODO.8377852 (Gardner, 2024).
The supplement related to this article is available online at: https://doi.org/10.5194/esurf-12-581-2024-supplement.
AN and SC devised the project, the main conceptual ideas, and proof outline. JRG developed the SSC database. All authors discussed the results and contributed to the final manuscript.
At least one of the (co-)authors is a member of the editorial board of Earth Surface Dynamics. The peer-review process was guided by an independent editor, and the authors also have no other competing interests to declare.
Publisher's note: Copernicus Publications remains neutral with regard to jurisdictional claims made in the text, published maps, institutional affiliations, or any other geographical representation in this paper. While Copernicus Publications makes every effort to include appropriate place names, the final responsibility lies with the authors.
Sagy Cohen was partly funded by the National Oceanic and Atmospheric Administration (NOAA) through the Cooperative Institute for Research to Operations in Hydrology (CIROH), award no. A22-0305. John R. Gardner was funded by NASA-NIP no. 80NSSC21K0921.
This paper was edited by Joris Eekhout and reviewed by Otavio Montanher and Dongfeng Li.
Alemohammad, H. and Booth, K.: LandCoverNet: A global benchmark land cover classification training dataset (Version 1), arXiv [preprint], arXiv:2012.03111, https://doi.org/10.48550/ARXIV.2012.03111, 2020.
Alewell, C., Borrelli, P., Meusburger, K., and Panagos, P.: Using the USLE: Chances, challenges and limitations of soil erosion modelling, Int. Soil Water Conserv. Res., 7, 203–225, https://doi.org/10.1016/j.iswcr.2019.05.004, 2019.
Altenau, E. H., Pavelsky, T. M., Durand, M. T., Yang, X., Frasson, R. P. D. M., and Bendezu, L.; SWOT River Database (SWORD) (Version v1), Zenodo [data set], https://doi.org/10.5281/ZENODO.4917236, 2021.
American Academy of Actuaries: Actuaries Climate Index Sample Calculations, https://actuariesclimateindex.org/wp-content/uploads/2016/04/SampleCalcEng.5.18.pdf (last access: 25 April 2024), 2016.
Armijos, E., Crave, A., Espinoza, J. C., Filizola, N., Espinoza-Villar, R., Ayes, I., Fonseca, P., Fraizy, P., Gutierrez, O., Vauchel, P., Camenen, B., Martiínez, J. M., Dos Santos, A., Santini, W., Cochonneau, G., and Guyot, J. L.: Rainfall control on Amazon sediment flux: synthesis from 20 years of monitoring, Environ. Res. Commun., 2, 5, https://doi.org/10.1088/2515-7620/ab9003, 2020.
Ayes Rivera, I., Molina-Carpio, J., Espinoza, J. C., Gutierrez-Cori, O., Cerón, W. L., Frappart, F., Armijos Cardenas, E., Espinoza-Villar, R., Ayala, J. M., and Filizola, N.: The Role of the Rainfall Variability in the Decline of the Surface Suspended Sediment in the Upper Madeira Basin (2003–2017), Front. Water, 3, 738527, https://doi.org/10.3389/frwa.2021.738527, 2021.
Barthem, R., Marques, M., Charvet, P., and Montag, L.: Amazon River Basin: I – Characterization and Environmental Impacts due to Deforestation, WIT Trans. Ecol. Environ., 81, 615–625, 2005.
Blöschl, G., Ardoin-Bardin, S., Bonell, M., Dorninger, M., Goodrich, D., Gutknecht, D., Matamoros, D., Merz, B., Shand, P., and Szolgay, J.: At what scales do climate variability and land cover change impact on flooding and low flows?, Hydrol. Process, 21, 1241–1247, https://doi.org/10.1002/hyp.6669, 2007.
Breil, M., Davin, E. L., and Rechid, D.: What determines the sign of the evapotranspiration response to afforestation in European summer?, Biogeosciences, 18, 1499–1510, https://doi.org/10.5194/bg-18-1499-2021, 2021.
Bringhurst, K. and Jordan, P.: The impact on nutrient cycles from tropical forest to pasture conversion in Costa Rica, Sustain. Water Resour. Manage., 1, 3–13, https://doi.org/10.1007/s40899-015-0003-x, 2015.
Broadbent, E. N., Asner, G. P., Keller, M., Knapp, D. E., Oliveira, P. J. C., and Silva, J. N.: Forest fragmentation and edge effects from deforestation and selective logging in the Brazilian Amazon, Biol. Conserv., 141, 1745–1757, https://doi.org/10.1016/j.biocon.2008.04.024, 2008.
Callède, J., Cochonneau, G., Alves, F., Guyot, J. L., Guimarães, V., and De Oliveira, E.: Les apports en eau de l'Amazone à l'Océan Atlantique, Rev. Sci. Eau, 23, 247–273, https://doi.org/10.7202/044688ar, 2010.
Cardelús, C. L., Mekonnen, A. B., Jensen, K. H., Woods, C. L., Baez, M. C., Montufar, M., Bazany, K., Tsegay, B. A., Scull, P. R., and Peck, W. H.: Edge effects and human disturbance influence soil physical and chemical properties in Sacred Church Forests in Ethiopia, Plant Soil, 453, 329–342, https://doi.org/10.1007/s11104-020-04595-0, 2020.
Chen, D., Huang, H., Hu, M., and Dahlgren, R. A.: Influence of Lag Effect, Soil Release, And Climate Change on Watershed Anthropogenic Nitrogen Inputs and Riverine Export Dynamics, Environ. Sci. Technol., 48, 5683–5690, https://doi.org/10.1021/es500127t, 2014.
Climate Hazard Group: CHIRPS: Quasi-global daily satellite and observation based precipitation estimates over land, Climate Hazard Group [data set], https://catalogue.ceda.ac.uk/uuid/4e53c2aee3fe44e7aa107c163696d2e7 (last access: 10 March 2022), 2018.
Coe, M. T., Costa, M. H., and Soares-Filho, B. S.: The influence of historical and potential future deforestation on the stream flow of the Amazon River – Land surface processes and atmospheric feedbacks, J. Hydrol., 369, 165–174, https://doi.org/10.1016/j.jhydrol.2009.02.043, 2009.
Coe, M. T., Latrubesse, E. M., Ferreira, M. E., and Amsler, M. L.: The effects of deforestation and climate variability on the streamflow of the Araguaia River, Brazil, Biogeochemistry, 105, 119–131, https://doi.org/10.1007/s10533-011-9582-2, 2011.
Costa, M. H., Botta, A., and Cardille, J. A.: Effects of large-scale changes in land cover on the discharge of the Tocantins River, Southeastern Amazonia, J. Hydrol., 283, 206–217, https://doi.org/10.1016/s0022-1694(03)00267-1, 2003.
Costa, M. H., Coe, M. T., and Loup Guyot, J.: Effects of climatic variability and deforestation on surface water regimes, Amazonia and Global Change, American Geophysical Union, 543–553, https://doi.org/10.1029/2008gm000738, 2009.
Crochemore, L., Isberg, K., Pimentel, R., Pineda, L., Hasan, A., and Arheimer, B., Lessons learnt from checking the quality of openly accessible river flow data worldwide, Hydrolog. Sci. J., 65, 699–711, https://doi.org/10.1080/02626667.2019.1659509, 2019.
D'Almeida, C., Vörösmarty, C. J., Marengo, J. A., Hurtt, G. C., Dingman, S. L., and Keim, B. D.: A water balance model to study the hydrological response to different scenarios of deforestation in Amazonia, J. Hydrol., 331, 125–136, https://doi.org/10.1016/j.jhydrol.2006.05.027, 2006.
da Cruz, D. C., Benayas, J. M. R., Ferreira, G. C., Santos, S. R., and Schwartz, G.: An overview of forest loss and restoration in the Brazilian Amazon, New Forests, 52, 1–16, https://doi.org/10.1007/s11056-020-09777-3, 2020.
Deforestation in the Amazon: https://rainforests.mongabay.com/amazon/amazon_destruction.html (last access: 3 April 2022), 2021.
Dethier, E. N., Renshaw, C. E., and Magilligan, F. J.: Toward Improved Accuracy of Remote Sensing Approaches for Quantifying Suspended Sediment: Implications for Suspended-Sediment Monitoring, J. Geophys. Res.-Earth, 125, e2019JF005033, https://doi.org/10.1029/2019jf005033, 2020.
Dethier, E. N., Renshaw, C. E., and Magilligan, F. J.: Rapid changes to global river suspended sediment flux by humans, Science, 376, 1447–1452, https://doi.org/10.1126/science.abn7980, 2022.
Diringer, S. E., Berky, A. J., Marani, M., Ortiz, E. J., Karatum, O., Plata, D. L., Pan, W. K., and Hsu-Kim, H.: Deforestation Due to Artisanal and Small-Scale Gold Mining Exacerbates Soil and Mercury Mobilization in Madre de Dios, Peru, Environ. Sci. Technol., 54, 286–296, https://doi.org/10.1021/acs.est.9b06620, 2019.
Durin, B., Plantak, L., Bonacci, O., and Di Nunno, F.: A Unique Approach to Hydrological Behavior along the Bednja River (Croatia) Watercourse, Water, 15, 589, https://doi.org/10.3390/w15030589, 2023.
Dykes, A. P.: Rainfall interception from a lowland tropical rainforest in Brunei, J. Hydrol., 200, 260–279, https://doi.org/10.1016/s0022-1694(97)00023-1, 1997.
Ellison, D., Futter, M. N., and Bishop, K.: On the forest cover–water yield debate: from demand- to supply-side thinking, Global Change Biol., 18, 806–820, https://doi.org/10.1111/j.1365-2486.2011.02589.x, 2011.
Ellison, D., Morris, C. E., Locatelli, B., Sheil, D., Cohen, J., Murdiyarso, D., Gutierrez, V., van Noordwijk, M., Creed, I. F., Pokorny, J., Gaveau, D., Spracklen, D. V., Tobella, A. B., Ilstedt, U., Teuling, A. J., Gebrehiwot, S. G., Sands, D. C., Muys, B., Verbist, B., Springgay, E., Sugandi, Y., and Sullivan, C. A.: Trees, forests and water: Cool insights for a hot world, Global Environ. Change, 43, 51–61, https://doi.org/10.1016/j.gloenvcha.2017.01.002, 2017.
Espinoza, J. C., Chavez, S., Ronchail, J., Junquas, C., Takahashi, K., and Lavado, W.: Rainfall hotspots over the southern tropical Andes: Spatial distribution, rainfall intensity, and relations with large-scale atmospheric circulation, Water Resour. Res., 51, 3459–3475, https://doi.org/10.1002/2014wr016273, 2015.
Fitzpatrick, F. A., and Knox, J. C.: Spatial and temporal sensitivity of hydrogeomorphic responce and recovery to deforestation, Agriculture, and floods, Phys. Geogr., 21, 89–108, https://doi.org/10.1080/02723646.2000.10642701, 2000.
Flores, B. M., Staal, A., Jakovac, C. C., Hirota, M., Holmgren, M., and Oliveira, R. S.: Soil erosion as a resilience drain in disturbed tropical forests, Plant Soil., 450, 11–25, https://doi.org/10.1007/s11104-019-04097-8, 2019.
Foley, J. A., Asner, G. P., Costa, M. H., Coe, M. T., DeFries, R., Gibbs, H. K., Howard, E. A., Olson, S., Patz, J., Ramankutty, N., and Snyder, P.: Amazonia revealed: forest degradation and loss of ecosystem goods and services in the Amazon Basin, Front. Ecol. Environ., 5, 25–32, https://doi.org/10.1890/1540-9295(2007)5[25:ARFDAL]2.0.CO;2, 2007.
Funk, C., Peterson, P., Landsfeld, M., Pedreros, D., Verdin, J., Shukla, S., Husak, G., Rowland, J., Harrison, L., Hoell, A., and Michaelsen, J.: The climate hazards infrared precipitation with stations – a new environmental record for monitoring extremes, Sci. Data, 2, 1, https://doi.org/10.1038/sdata.2015.66, 2015.
Gardner, J.: River Sediment Database-Amazon (RivSed-Amazon) (v1.1), Zenodo [data set and code], https://doi.org/10.5281/ZENODO.8377852, 2024.
Gardner, J., Pavelsky, T., Topp, S., Yang, X., Ross, M. R. V., and Cohen, S.: Human activities change suspended sediment concentration along rivers, Environ. Res. Lett., 18, 064032, https://doi.org/10.1088/1748-9326/acd8d8, 2023.
Gardner, J. R., Yang, X., Topp, S. N., Ross, M. R. V., Altenau, E. H., and Pavelsky, T. M.: The Color of Rivers, Geophys. Res. Lett., 48, e2020GL088946, https://doi.org/10.1029/2020gl088946, 2021.
Guzha, A. C., Rufino, M. C., Okoth, S., Jacobs, S., and Nóbrega, R. L. B.: Impacts of land use and land cover change on surface runoff, discharge and low flows: Evidence from East Africa, Hydrol. Reg. Stud., 15, 49–67, https://doi.org/10.1016/j.ejrh.2017.11.005, 2018.
Hansen, M. C., Potapov, P. V., Moore, R., Hancher, M., Turubanova, S. A., Tyukavina, A., Thau, D., Stehman, S. V., Goetz, S. J., Loveland, T. R., Kommareddy, A., Egorov, A., Chini, L., Justice, C. O., and Townshend, J. R. G.: High-Resolution Global Maps of 21st-Century Forest Cover Change, Science, 342, 850–853, https://doi.org/10.1126/science.1244693, 2013.
Horton, A. J., Constantine, J. A., Hales, T. C., Goossens, B., Bruford, M. W., and Lazarus, E. D.: Modification of river meandering by tropical deforestation, Geology, 45, 511–514, https://doi.org/10.1130/g38740.1, 2017.
Ilstedt, U., Malmer, A., Verbeeten, E., and Murdiyarso, D.: The effect of afforestation on water infiltration in the tropics: A systematic review and meta-analysis, Forest Ecol. Manage., 251, 45–51, https://doi.org/10.1016/j.foreco.2007.06.014, 2007.
Institut national des sciences de l'Univers: HYdro-géochimie du Bassin Amazonien, SO-HYBAM, https://hybam.obs-mip.fr/ (last access: 26 April 2024), 2021.
Instituto Nacional de Pesquisas Espaciais: Measurement of Deforestation by Remote Sensing, PRODES, http://terrabrasilis.dpi.inpe.br/downloads (last access: 26 April 2024), 2020.
Kettner, A. J., Gomez, B., and Syvitski, J. P. M.: Modeling suspended sediment discharge from the Waipaoa River system, New Zealand: The last 3000 years, Water Resour. Res., 43, W07411, https://doi.org/10.1029/2006wr005570, 2007.
Kovacic, G., and Nataša Ravbar, N.: Extreme hydrological events in karst areas of Slovenia, the case of the Unica River basin, Geodin. Acta, 23, 89–100, https://doi.org/10.3166/ga.23.89-100, 2010.
Kreiling, R. M., Thoms, M. C., Bartsch, L. A., Larson, J. H., and Christensen, V. G.: Land Use Effects on Sediment Nutrient Processes in a Heavily Modified Watershed Using Structural Equation Models, Water Resour. Res., 56, e2019WR026655, https://doi.org/10.1029/2019wr026655, 2020.
Kroese, J. S., Jacobs, S. R., Tych, W., Breuer, L., Quinton, J. N., and Rufino, M. C.: Tropical Montane Forest Conversion Is a Critical Driver for Sediment Supply in East African Catchments, Water Resour. Res., 56, e2020WR02749, https://doi.org/10.1029/2020wr027495, 2020.
Lal, R.: Deforestation effects on soil degradation and rehabilitation in western Nigeria. IV. Hydrology and water quality, Land Degrad. Dev., 8, 95–126, https://doi.org/10.1002/(sici)1099-145x(199706)8:2<95::aid-ldr241>3.0.co;2-k, 1997.
Latrubesse, E. M., Amsler, M. L., de Morais, R. P., and Aquino, S.: The geomorphologic response of a large pristine alluvial river to tremendous deforestation in the South American tropics: The case of the Araguaia River, Geomorphology, 113, 239–252, https://doi.org/10.1016/j.geomorph.2009.03.014, 2009.
Lehner, B. and Grill, G.: Global river hydrography and network routing: Baseline data and new approaches to study the world's large river systems, Hydrol. Process., 27, 2171–2186, https://doi.org/10.1002/hyp.9740, 2013.
Lucas-Borja, M. E., Carrà, B. G., Nunes, J. P., Bernard-Jannin, L., Zema, D. A., and Zimbone, S. M.: Impacts of land-use and climate changes on surface runoff in a tropical forest watershed (Brazil), Hydrolog. Sci. J., 65, 1956–1973, https://doi.org/10.1080/02626667.2020.1787417, 2020.
Maeda, E. E., Formaggio, A. R., and Shimabukuro, Y. E.: Impacts of Land Use and Land Cover Changes on Sediment Yield in a Brazilian Amazon Drainage Basin, GISci. Remote Sens., 45, 443–453, https://doi.org/10.2747/1548-1603.45.4.443, 2008.
Maina, J., de Moel, H., Zinke, J., Madin, J., McClanahan, T., and Vermaat, J. E.: Human deforestation outweighs future climate change impacts of sedimentation on coral reefs, Nat. Commun., 4, 1986, https://doi.org/10.1038/ncomms2986, 2013.
Marconcini, M., Metz-Marconcini, A., Üreyen, S., Palacios-Lopez, D., Hanke, W., Bachofer, F., Zeidler, J., Esch, T., Gorelick, N., Kakarla, A., Paganini, M., and Strano, E.: Outlining where humans live, the World Settlement Footprint 2015, Sci. Data, 7, 1, https://doi.org/10.1038/s41597-020-00580-5, 2020.
Meals, D. W. and Dressing, S. A.: Lag time in water quality response to land treatment, https://www.epa.gov/polluted-runoffnonpoint-source-pollution/nonpoint-source-monitoring-technical-notes (last access: 7 November 2023), 2008.
Meyer, H., Reudenbach, C., Hengl, T., Katurji, M., and Nauss, T.: Improving performance of spatio-temporal machine learning models using forward feature selection and target-oriented validation, Environ. Model. Softw., 101, 1–9. https://doi.org/10.1016/j.envsoft.2017.12.001, 2018.
Milliman, J. D. and Farnsworth, K. L.: River discharge to the coastal ocean: a global synthesis, Cambridge University Press, ISBN 978-0-521-87987-3, 2011.
Moges, E., Demissie, Y., Larsen, L., and Yassin, F., Review: Sources of Hydrological Model Uncertainties and Advances in Their Analysis, Water, 13, 28, https://doi.org/10.3390/w13010028, 2020.
Montanher, O. C., Novo, E. M. L. M., Barbosa, C. C. F., Rennó, C. D., and Silva, T. S. F.: Empirical models for estimating the suspended sediment concentration in Amazonian white water rivers using Landsat 5/TM, Int. J. Appl. Earth Obs. Geoinf., 29, 67–77, https://doi.org/10.1016/j.jag.2014.01.001, 2014.
Moragoda, N., Cohen, S., Gardner, J., Muñoz, D., Narayanan, A., Moftakhari, H., and Pavelsky, T. M.: Modeling and Analysis of Sediment Trapping Efficiency of Large Dams Using Remote Sensing, Water Resour. Res., 59, e2022WR033296, https://doi.org/10.1029/2022wr033296, 2023.
Ochiai, S., Nagao, S., Yonebayashi, K., Fukuyama, T., Suzuki, T., Yamamoto, M., and Nakamura, K.: Effect of deforestation on the transport of particulate organic matter inferred from the geochemical properties of reservoir sediments in the Noto Peninsula, Japan, Geochem. J., 49, 513–522, https://doi.org/10.2343/geochemj.2.0379, 2015.
Ouyang, Y., Leininger, T. D., and Moran, M.: Impacts of reforestation upon sediment load and water outflow in the Lower Yazoo River Watershed, Mississippi, Ecol. Eng., 61, 394–406, https://doi.org/10.1016/j.ecoleng.2013.09.057, 2013.
Owens, P. N., Petticrew, E. L., and van der Perk, M.: Sediment response to catchment disturbances. J. Soils Sediments, 10, 591–596, https://doi.org/10.1007/s11368-010-0235-1, 2010.
Potić, I., Mihajlović, L. M., Šimunić, V., Ćurčić, N. B., and Milinčić, M.: Deforestation as a Cause of Increased Surface Runoff in the Catchment: Remote Sensing and SWAT Approach – A Case Study of Southern Serbia, Front. Environ. Sci., 10, 896404, https://doi.org/10.3389/fenvs.2022.896404, 2022.
Pruett, T. S.: Rethinking the Economic Geography of the Coca Leaf: Fortune, Folly, or Fantasy?, Focus Geogr., 57, 84–96, https://doi.org/10.1111/foge.12030, 2014.
Renard, K. G.: Predicting soil erosion by water: a guide to conservation planning with the Revised Universal Soil Loss Equation (RUSLE), United States Government Printing, https://www.ars.usda.gov/arsuserfiles/64080530/rusle/ah_703.pdf (last access: 19 March 2022), 1997.
Restrepo, J., Kettner, A., and Syvitski, J.: Recent deforestation causes rapid increase in river sediment load in the Colombian Andes, Anthropocene, 10, 13–28, https://doi.org/10.1016/j.ancene.2015.09.001, 2015.
Reubens, B., Poesen, J., Danjon, F., Geudens, G., and Muys, B.: The role of fine and coarse roots in shallow slope stability and soil erosion control with a focus on root system architecture: a review, Trees, 21, 385–402, https://doi.org/10.1007/s00468-007-0132-4, 2007.
Rodriguez, D. A., Tomasella, J., and Linhares, C.: Is the forest conversion to pasture affecting the hydrological response of Amazonian catchments? Signals in the Ji-Paraná Basin, Hydrol. Process., 24, 1254–1269, https://doi.org/10.1002/hyp.7586, 2010.
Rosa, I. M., Smith, M. J., Wearn, O. R., Purves, D., and Ewers, R. M.: The Environmental Legacy of Modern Tropical Deforestation, Curr. Biol., 26, 2161–2166, https://doi.org/10.1016/j.cub.2016.06.013, 2016.
Ross, M. R., Topp, S. N., Appling, A. P., Yang, X., Kuhn, C., Butman, D., Simard, M., and Pavelsky, T. M.; AquaSat: A data set to enable remote sensing of water quality for inland waters, Water Resour. Res., 55, 10012–10025, https://doi.org/10.1029/2019WR024883, 2019.
Seegers, B. N., Stumpf, R. P., Schaeffer, B. A., Loftin, K. A., and Werdell, P. J.: Performance metrics for the assessment of satellite data products: an ocean color case study, Opt. Express, 26, 7404, https://doi.org/10.1364/oe.26.007404, 2018.
Shimada, M., Itoh, T., Motooka, T., Watanabe, M., Shiraishi, T., Thapa, R., and Lucas, R.: New global forest/non-forest maps from ALOS PALSAR data (2007–2010), Remote Sens. Environ., 155, 13–31, https://doi.org/10.1016/j.rse.2014.04.014, 2014.
Silva Junior, C. H. L., Pessôa, A. C. M., Carvalho, N. S., Reis, J. B. C., Anderson, L. O., and Aragão, L. E. O. C.: The Brazilian Amazon deforestation rate in 2020 is the greatest of the decade, Nat. Ecol. Evol., 5, 144–145, https://doi.org/10.1038/s41559-020-01368-x, 2020.
Souza Jr., C. M., Shimbo, Z. J., Rosa, M. R., Parente, L. L., Alencar, A., Rudorff, B. F. T., Hasenack, H., Matsumoto, M. G., Ferreira, L., Souza-Filho, P. W. M., de Oliveira, S. W., Rocha, W. F., Fonseca, A. V., Marques, C. B., Diniz, C. G., Costa, D., Monteiro, D., Rosa, E. R., Vélez-Martin, E., Weber, E. J., Lenti, F. E. B., Paternost, F. F., Pareyn, F. G. C., Siqueira, J. V., Viera, J. L., Neto, L. C. F., Saraiva, M. M., Sales, M. H., Salgado, M. P. G., Vasconcelos, R., Galano, S., Mesquita, V. V., and Azevedo, T.: Reconstructing Three Decades of Land Use and Land Cover Changes in Brazilian Biomes with Landsat Archive and Earth Engine, Remote Sens., 12, 2735 https://doi.org/10.3390/rs12172735, 2020.
Sweeney, B. W., Bott, T. L., Jackson, J. K., Kaplan, L. A., Newbold, J. D., Standley, L. J., Hession, W. C., and Horwitz, R. J.: Riparian deforestation, stream narrowing, and loss of stream ecosystem services, P. Natl. Acad. Sci. USA, 101, 4132–14137, https://doi.org/10.1073/pnas.0405895101, 2004.
Talib, A. and Randhir, T. O.: Long-term effects of land-use change on water resources in urbanizing watersheds, PloS Water, 2, e0000083, https://doi.org/10.1371/journal.pwat.0000083, 2023.
Van Dun, M. E. H.: Cocaleros. Violence, drugs and social mobilization in the post-conflict Upper Huallaga Valley, Peru, Rozenberg Publishers, ISBN 978-90-361-0120-2, 2009.
Veldkamp, E., Schmidt, M., Powers, J. S., and Corre, M. D.: Deforestation and reforestation impacts on soils in the tropics, Nat. Rev. Earth Environ., 1, 590–605, https://doi.org/10.1038/s43017-020-0091-5, 2020.
Venticinque, E., Forsberg, B., Barthem, R., Petry, P., Hess, L., Mercado, A., Cañas, C., Montoya, M., Durigan, C., and Goulding, M.: An explicit GIS-based river basin framework for aquatic ecosystem conservation in the Amazon, Earth Syst. Sci. Data, 8, 651–661, https://doi.org/10.5194/essd-8-651-2016, 2016a.
Venticinque, E., Forsberg, B., B. Barthem, R., Petry, P., Hass, L., Mercado, A., Canas, C., Montoya, M., Durigan, C., and Goulding, M.: SNAPP Western Amazon Group – Amazon Aquatic Ecosystem Spatial Framework, KNB Data Repository [data set], https://doi.org/10.5063/F1BG2KX8, 2016b.
Voldoire, A. and Royer, J. F.: Tropical deforestation and climate variability, Clim. Dynam., 22, 857–874, https://doi.org/10.1007/s00382-004-0423-z, 2004.
Walling, D. E.: The sediment delivery problem, J. Hydrol., 65, 209–237, https://doi.org/10.1016/0022-1694(83)90217-2, 1983.
Walling, D. E.: Linking land use, erosion and sediment yields in river basins, in: Man and River Systems. Developments in Hydrobiology, 146, edited by: Garnier J. and Mouchel, J. M., Springer, 223–240, https://doi.org/10.1007/978-94-017-2163-9_24, 1999.
Wang, S., Fu, B., Piao, S., Lü, Y, Philippe, C., Feng, X., and Wang, Y.: Reduced sediment transport in the Yellow River due to anthropogenic changes, Nat. Geosci., 9, 38–41, https://doi.org/10.1038/ngeo2602, 2016.
Wasson, R. J., Juyal, N., Jaiswal, M., McCulloch, M., Sarin, M. M., Jain, V., Srivastava, P., and Singhvi, A. K.: The mountain-lowland debate: deforestation and sediment transport in the upper Ganga catchment, J. Environ. Manage., 88, 53–61 https://doi.org/10.1016/j.jenvman.2007.01.046, 2008.
Water Resources National Agency (ANA): Brazilian Water Resources Database, https://www.snirh.gov.br/hidroweb/mapa (last access: 25 April 2024), 2020.
Wei, W., Chen, L., Fu, B., Lü, Y., and Gong, J.: Responses of water erosion to rainfall extremes and vegetation types in a loess semiarid hilly area, NW China, Hydrol. Process., 23, 1780–1791, https://doi.org/10.1002/hyp.7294, 2009.
Wei, W., Chen, L., Zhang, H., and Chen, J.: Effect of rainfall variation and landscape change on runoff and sediment yield from a loess hilly catchment in China, Environ. Earth Sci., 73, 1005–1016, https://doi.org/10.1007/s12665-014-3451-y, 2014.
Xing, F., Kettner, A. J., Ashton, A., Giosan, L., Ibáñez, C., and Kaplan, J. O.: Fluvial response to climate variations and anthropogenic perturbations for the Ebro River, Spain in the last 4000 years, Sci. Total Environ., 473–474, 20–31, hhttps://doi.org/10.1016/j.scitotenv.2013.11.083, 2014.
Yang, Z., Xi, W., Yang, Z., Shi, Z., Huang, G., Guo, J., and Yang, D.: Time-Lag Response of Landslide to Reservoir Water Level Fluctuations during the Storage Period: A Case Study of Baihetan Reservoir, Water, 15, 2732, https://doi.org/10.3390/w15152732, 2023.
Yepez, S., Laraque, A., Martinez, J.-M., De Sa, J., Carrera, J. M., Castellanos, B., Gallay, M., and Lopez, J. L.: Retrieval of suspended sediment concentrations using Landsat-8 OLI satellite images in the Orinoco River (Venezuela), CR Geosci., 350, 20–30, https://doi.org/10.1016/j.crte.2017.08.004, 2018.
Zeilhofer, P., Hallak Alcantara, L., and Fantim-Cruz, I.: Effects of Deforestation on Spatio-Temporal Runoff Patterns in the Upper Teles Pires Watershed, Mato Grosso, Brazil, Rev. Bras. Geogr. Fís., 11, , 1889–1901, 2018.
Zhang, M., Liu, N., Harper, R., Li, Q., Liu, K., Wei, X., Ning, D., Hou, Y., and Liu, S.: A global review on hydrological responses to forest change across multiple spatial scales: Importance of scale, climate, forest type and hydrological regime, J. Hydrol, 546, 44–59, https://doi.org/10.1016/j.jhydrol.2016.12.040, 2017.
Zhang, Z., Sheng, L., Yang, J., Chen, X. A., Kong, L., and Wagan, B.: Effects of Land Use and Slope Gradient on Soil Erosion in a Red Soil Hilly Watershed of Southern China, Sustainability, 7, 14309–14325, https://doi.org/10.3390/su71014309, 2015.
Zhao, B., Lei, H., Yang, D., Yang, S., and Santisirisomboon, J.: Runoff and sediment response to deforestation in a large Southeast Asian monsoon watershed, J. Hydrol., 606, 127432, https://doi.org/10.1016/j.jhydrol.2022.127432, 2022.
Zhou, Y., Li, D., Lu, J., Yao, S., Yan, X., Jin, Z., Liu, L., and Lu, X. X.: Distinguishing the multiple controls on the decreased sediment flux in the Jialing River basin of the Yangtze River, Southwestern China, Catena, 193, 104593, https://doi.org/10.1016/j.catena.2020.104593, 2020.
This study investigates the profound impact of deforestation in the Amazon on sediment dynamics. Novel remote sensing data and statistical analyses reveal significant changes, especially in heavily deforested regions, with rapid effects within a year. In less disturbed areas, a 1- to 2-year lag occurs, influenced by natural sediment shifts and human activities. These findings highlight the need to understand the consequences of human activity for our planet's future.
This study investigates the profound impact of deforestation in the Amazon on sediment dynamics....